Variation
is a problem for speech perception
[Newman, Clouse, & Burnham, 2001]
[Newman, Clouse, & Burnham, 2001]
An unfamiliar talker appears!
Listeners have to simultaneously infer what the talker is saying and how they say things
An ideal adpater rapidly adapts to an unfamiliar talker via distributional learning
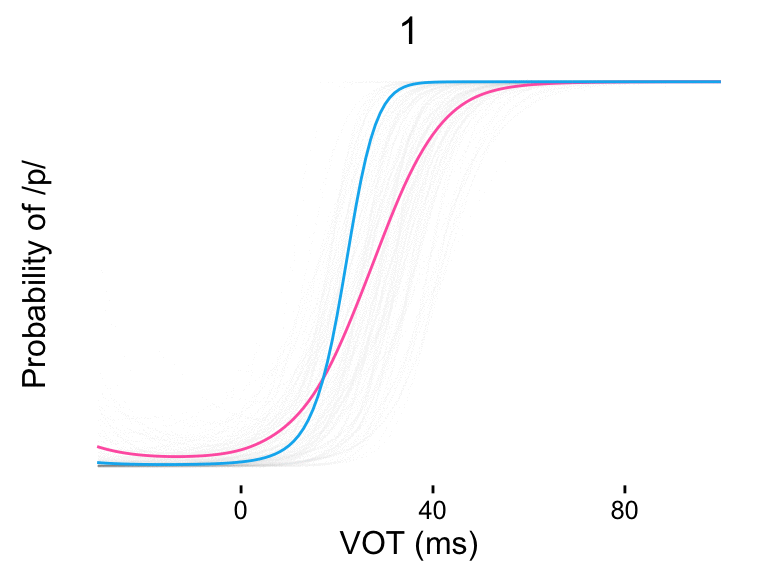
[Kleinschmidt & Jaeger, 2015]
Distributional learning needs to start somewhere
(prior beliefs in Bayesian jargon)
Scylla and Charybdis
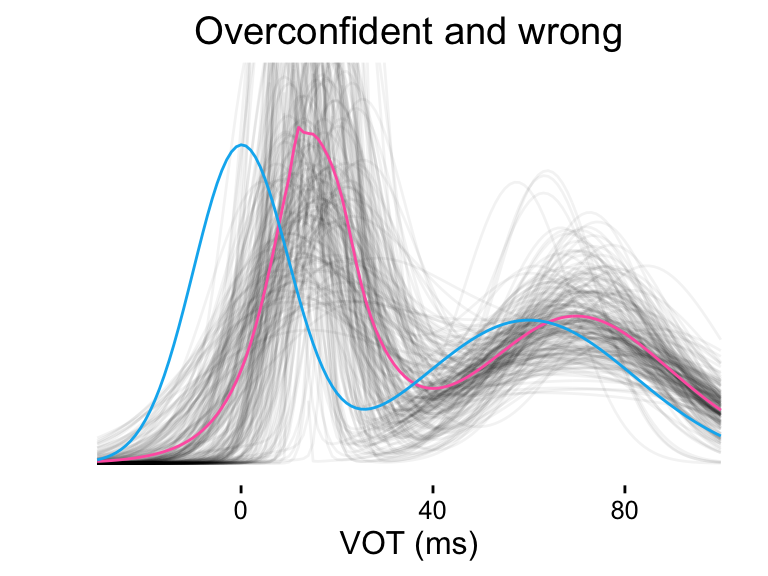
Too much uncertainty
Overconfident and wrong
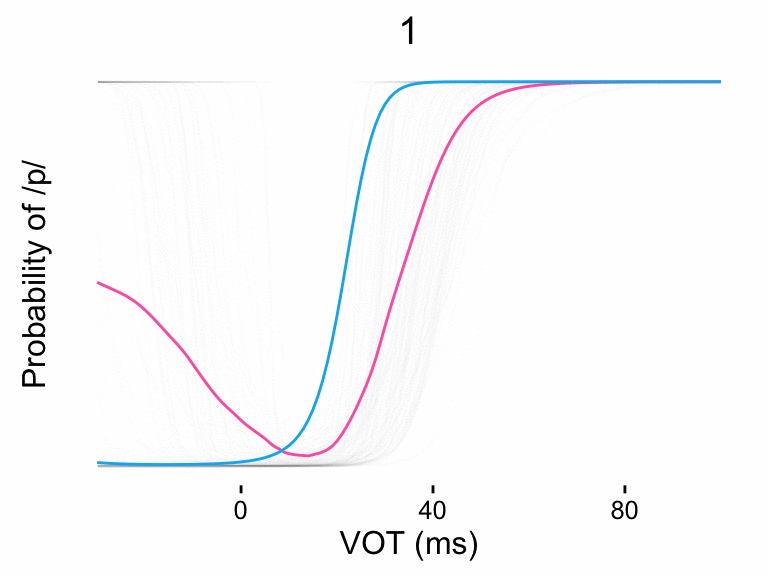
Ideal adapter predicts:
- Listeners’ expectations about an unfamiliar talkers should be as specific as possible while still being right on average.
- Adaptation is constrained by actual variability across talkers (as experienced by each listener).
- Accents that fall outside the range of normal variability should result in less adaptation.
Questions
- Is rapid adaptation to an unfamiliar talker constrained?
- Are constraints consistent with belief updating, starting from shared prior beliefs?
- Are these inferred prior beliefs ‘rational’ in that they reflect cross-talker variation?